MLflow
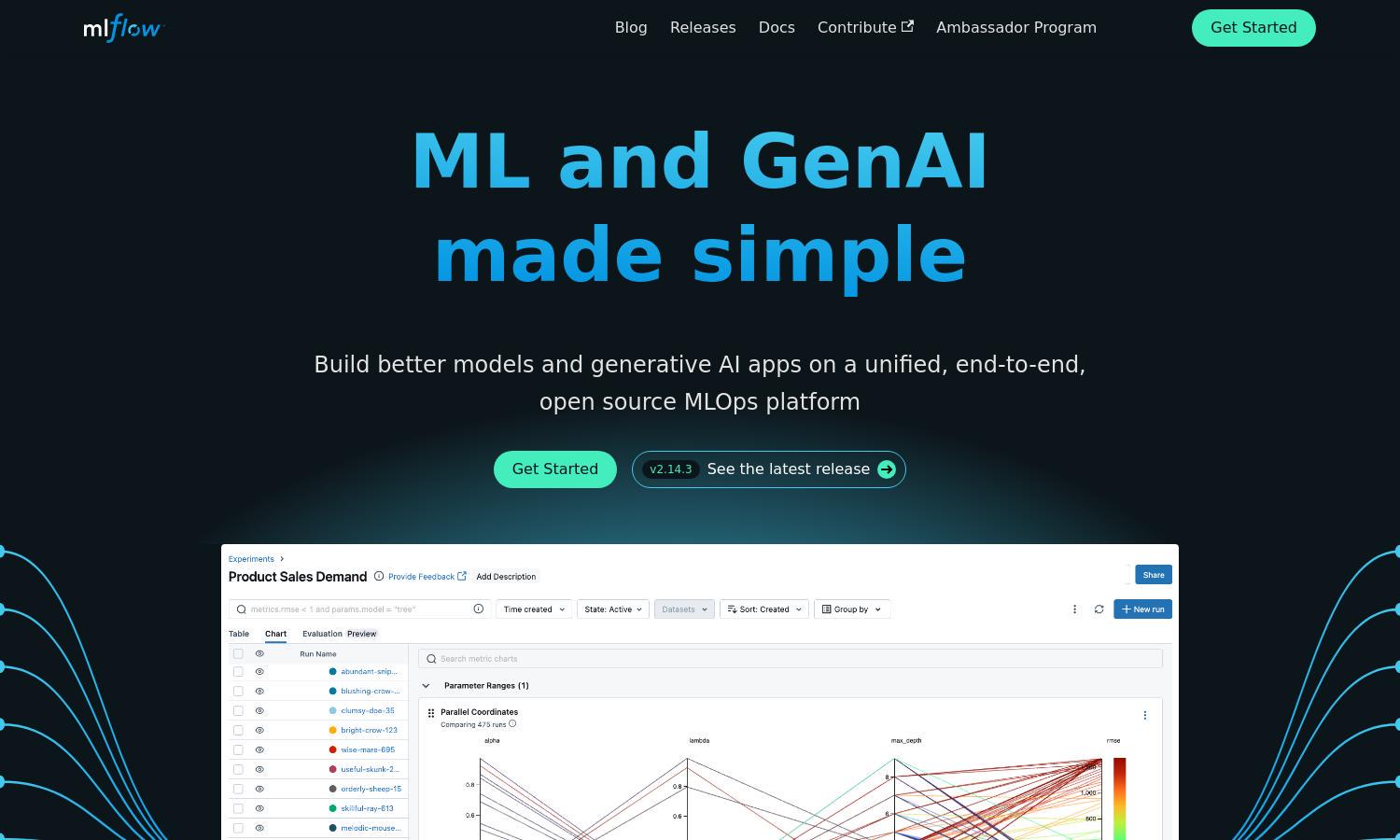
About MLflow
MLflow is a comprehensive, open-source platform for managing machine learning and generative AI projects. It facilitates experiment tracking, model deployment, and lifecycle management, addressing the challenges data scientists face. With a user-friendly interface and powerful features, MLflow enhances collaboration and efficiency in complex machine learning tasks.
MLflow offers a variety of user-friendly pricing plans, ensuring accessibility for all. The free tier provides essential features, while premium plans grant access to advanced tools and dedicated support. Upgrading unlocks enhanced functionality, facilitating improved model management and streamlined workflows, perfectly suiting the needs of diverse data practitioners.
MLflow's intuitive user interface ensures a smooth browsing experience, highlighted by dynamic dashboards and clear navigation. Users can easily track experiments and manage models through its streamlined layout. The thoughtful design of MLflow complements its powerful tools, empowering data scientists to focus on their projects with minimal hassle.
How MLflow works
Users begin their journey with MLflow by signing up and onboarding through simple instructions. The platform allows them to create experiments, log parameters, and track results effortlessly. As users navigate to the dashboard, they can visualize performance metrics, deploy models, and integrate with other tools, ensuring a seamless experience throughout the machine learning lifecycle.
Key Features for MLflow
Experiment Tracking
MLflow's Experiment Tracking feature allows users to efficiently log and compare experiment runs. This unique functionality lets data scientists analyze performance metrics, facilitating informed decisions while optimizing models. With MLflow, users can effortlessly track the entire lifecycle of their ML projects, driving better outcomes and collaboration.
Model Registry
The Model Registry in MLflow offers a centralized hub for managing and versioning machine learning models. This key feature ensures users can track model lineage, enabling easy deployment and collaboration among data scientists. MLflow’s Model Registry simplifies the model lifecycle, enhancing productivity and governance.
Unified Platform
MLflow serves as a unified platform for diverse machine learning applications, merging traditional ML with generative AI. This feature allows users to seamlessly manage workflows, fostering collaboration across teams. By integrating various tools and frameworks, MLflow enhances operational efficiency and project scalability.
FAQs for MLflow
What unique benefits does MLflow offer for managing machine learning workflows?
MLflow stands out by providing a comprehensive open-source platform that streamlines the management of machine learning workflows. Users benefit from its robust experiment tracking, model deployment capabilities, and integration with various ML libraries. This unique combination enhances collaboration and efficiency, allowing data scientists to focus on delivering quality results.
How does MLflow enhance collaboration in machine learning projects?
MLflow enhances collaboration by offering a centralized dashboard for tracking experiments and managing models. This feature enables team members to share insights and results effortlessly, ensuring everyone stays informed throughout the project lifecycle. By fostering communication and transparency, MLflow allows data science teams to work cohesively and optimize their outcomes.
What user experience improvements can be found in MLflow's interface?
MLflow offers significant user experience improvements through its intuitive interface, which simplifies navigation and enhances accessibility. Users can easily access important features like experiment tracking and model management, thanks to its organized layout. These improvements ensure that users can efficiently manage their projects without being hindered by complex processes.
What competitive advantage does MLflow provide in machine learning management?
MLflow's competitive advantage lies in its open-source nature and comprehensive integration capabilities with various machine learning frameworks. This versatility enables organizations to adopt MLflow across diverse environments while maintaining flexibility. Its powerful features, such as experiment tracking and model registry, empower users to navigate the complexities of machine learning with ease.
How does MLflow support users in deploying machine learning models?
MLflow supports users in deploying machine learning models through its streamlined deployment tools, which facilitate the transition from development to production. With options for serving models directly or integrating with various platforms, MLflow ensures users can effectively deploy their models while maintaining consistency and reliability throughout the process.
What key features help users benefit from MLflow’s functionalities?
MLflow's key features, such as experiment tracking, model registry, and easy integrations, significantly enhance user experience. By providing clear insights into model performance and enabling collaboration, MLflow allows users to manage their machine learning projects effectively, ensuring they can address challenges and achieve better outcomes throughout their workflows.